Real-Time Low-Cost Fault Detection System Placed in Non-Drive End of Motors Based on Neural Networks
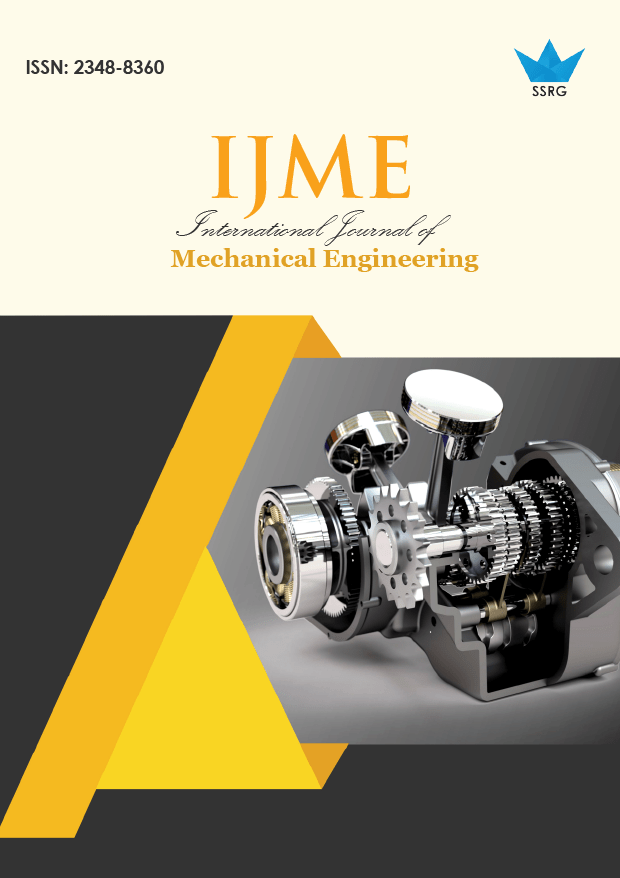
International Journal of Mechanical Engineering |
© 2024 by SSRG - IJME Journal |
Volume 11 Issue 8 |
Year of Publication : 2024 |
Authors : Brandon Borda Aliaga, Luis Florez Andia, Jesús Talavera S. |
How to Cite?
Brandon Borda Aliaga, Luis Florez Andia, Jesús Talavera S., "Real-Time Low-Cost Fault Detection System Placed in Non-Drive End of Motors Based on Neural Networks," SSRG International Journal of Mechanical Engineering, vol. 11, no. 8, pp. 134-140, 2024. Crossref, https://doi.org/10.14445/23488360/IJME-V11I8P115
Abstract:
Electric motors are vital components in various industrial applications, from production manufacturing to transportation and power generation. They are indispensable parts of machinery and equipment required for the industry, and their effective running is a must for maintaining operational efficiency and productivity. However, a motor suffers from different kinds of faults that can be expensive in terms of downtime, loss of production, and repair costs. Early detection of these faults is important in reducing unscheduled shutdowns, maintenance costs, and workplace accidents. This paper presents the design of a low-cost, real-time fault detection system for motors installed on the Non-Drive End based on neural networks, with the aim of enhancing operational efficiency and reducing maintenance costs in industries.
Keywords:
Non-Drive end, Neural network, Real-time detection, Low-cost, Fault Detection System.
References:
[1] J.R. Cameron, W.T. Thomson, and A.B. Dow, “Vibration and Current Monitoring for Detecting Airgap Eccentricity in Large Induction Motors,” IEE Proceedings B Electric Power Applications, vol. 133, no. 3, pp. 155-163, 1986.
[CrossRef] [Google Scholar] [Publisher Link]
[2] Peter Tavner et al., Condition Monitoring of Rotating Electrical Machines, London, U.K: IET, pp. 1-282, 2008.
[Google Scholar] [Publisher Link]
[3] Austin H. Bonnett, and Chuck Yung, “Increased Efficiency Versus Increased Reliability,” IEEE Industry Applications Magazine, vol. 14, no. 1, pp. 29-36, 2008.
[CrossRef] [Google Scholar] [Publisher Link]
[4] S. Nandi, H.A. Toliyat, and X. Li, “Condition Monitoring and Fault Diagnosis of Electrical Motors—A Review,” IEEE Transactions on Energy Conversion, vol. 20, no. 4, pp. 719-729, 2005.
[CrossRef] [Google Scholar] [Publisher Link]
[5] P.J. Tavner, “Review of Condition Monitoring of Rotating Electrical Machines,” IET Electric Power Applications, vol. 2, no. 4, pp. 215- 247, 2008.
[CrossRef] [Google Scholar] [Publisher Link]
[6] M. El Hachemi Benbouzid, “A Review of Induction Motors Signature Analysis as a Medium for Faults Detection,” IEEE Transactions on Industrial Electronics, vol. 47, no. 5, pp. 984-993, 2000.
[CrossRef] [Google Scholar] [Publisher Link]
[7] W.T. Thomson, and M. Fenger, “Current Signature Analysis to Detect Induction Motor Faults,” IEEE Industry Applications Magazine, vol. 7, no. 4, pp. 26-34, 2001.
[CrossRef] [Google Scholar] [Publisher Link]
[8] R. Beguenane, and M. El Hachemi Benbouzid, “Induction Motors Thermal Monitoring by Means of Rotor Resistance Identification,” IEEE International Electric Machines and Drives Conference Record, Milwaukee, WI, USA, pp. 4.1-4.3, 1997.
[CrossRef] [Google Scholar] [Publisher Link]
[9] Erinc Karatoprak, and Serhat Seker, “An Improved Empirical Mode Decomposition Method Using Variable Window Median Filter for Early Fault Detection in Electric Motors,” Mathematical Problems in Engineering, pp. 1-9, 2019.
[CrossRef] [Google Scholar] [Publisher Link]
[10] Ma’d El-Dalahmeh et al., “Autonomous Fault Detection and Diagnosis for Permanent Magnet Synchronous Motors Using Combined Variational Mode Decomposition, the Hilbert-Huang Transform, and a Convolutional Neural Network,” Computers and Electrical Engineering, vol. 110, pp. 1-22, 2023.
[CrossRef] [Google Scholar] [Publisher Link]
[11] P. Konar, and P. Chattopadhyay, “Bearing Fault Detection of Induction Motor Using Wavelet and Support Vector Machines (SVMs),” Applied Soft Computing, vol. 11, no. 6, pp. 4203-4211, 2011.
[CrossRef] [Google Scholar] [Publisher Link]
[12] Jing Tian et al., “Motor Bearing Fault Detection Using Spectral Kurtosis-Based Feature Extraction Coupled with K-Nearest Neighbor Distance Analysis,” IEEE Transactions on Industrial Electronics, vol. 63, no. 3, pp. 1793-1803, 2016.
[CrossRef] [Google Scholar] [Publisher Link]
[13] Long Wen et al., “A New Convolutional Neural Network-Based Data-Driven Fault Diagnosis Method,” IEEE Transactions on Industrial Electronics, vol. 65, no. 7, pp. 5990-5998, 2018.
[CrossRef] [Google Scholar] [Publisher Link]
[14] Dong-Jin Choi et al., “Comparative Study of CNN and RNN for Motor Fault Diagnosis Using Deep Learning,” IEEE 7th International Conference on Industrial Engineering and Applications,Bangkok, Thailand, pp. 693-696, 2020.
[CrossRef] [Google Scholar] [Publisher Link]
[15] Shrinathan Esaki Muthu Pandara Kone et al., “Application of Convolutional Neural Network for Fault Diagnosis of Bearing Scratch of an Induction Motor,” Applied Sciences, vol. 12, no. 11, pp. 1-13, 2022.
[CrossRef] [Google Scholar] [Publisher Link]
[16] Hyun Cheol Cho et al., “Fault Detection and Isolation of Induction Motors Using Recurrent Neural Networks and Dynamic Bayesian Modeling,” IEEE Transactions on Control Systems Technology, vol. 18, no. 2, pp. 430-437, 2010.
[CrossRef] [Google Scholar] [Publisher Link]
[17] Abhinav Saxena et al., “Abnormal Health Monitoring and Assessment of a Three-Phase Induction Motor Using a Supervised CNN-RNN-Based Machine Learning Algorithm,” Mathematical Problems in Engineering, vol. 2023, no. 1, pp. 1-8, 2023.
[CrossRef] [Google Scholar] [Publisher Link]
[18] Xinjie Shi et al., “Hybrid Convolutional Recurrent Neural Networks Outperform CNN and RNN in Task-state EEG Detection for Parkinson’s Disease,” Asia-Pacific Signal and Information Processing Association Annual Summit and Conference (APSIPA ASC), Lanzhou, China, pp. 939-944, 2019.
[CrossRef] [Google Scholar] [Publisher Link]
[19] Jyotibdha Acharya, and Arindam Basu, “Deep Neural Network for Respiratory Sound Classification in Wearable Devices Enabled by Patient Specific Model Tuning,” IEEE Transactions on Biomedical Circuits and Systems, vol. 14, no. 3, pp. 535-544, 2020.
[CrossRef] [Google Scholar] [Publisher Link]