Multi-Objective Optimization (MOO) of Machining Process Factors by Hybrid Taguchi Grey Relational Analysis (HGRA)
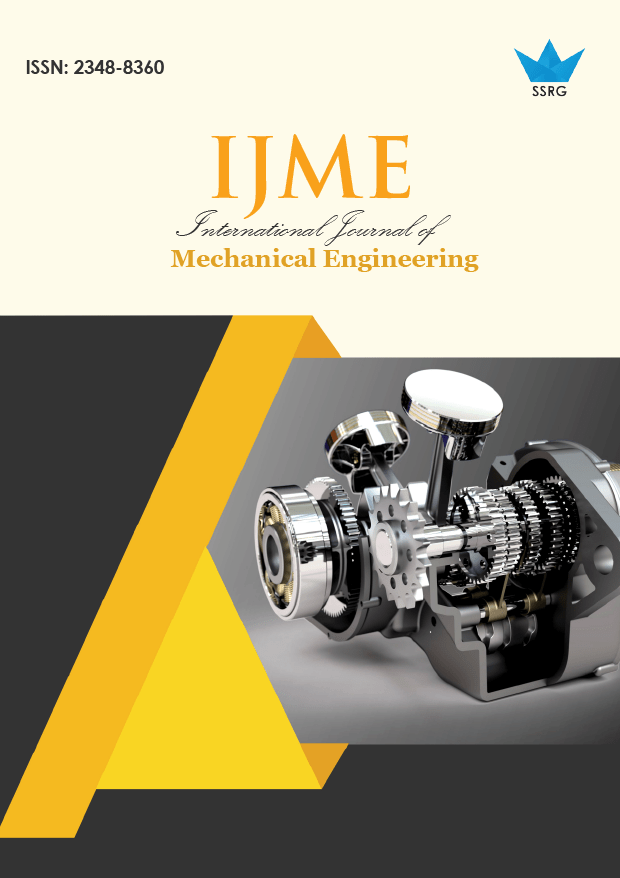
International Journal of Mechanical Engineering |
© 2025 by SSRG - IJME Journal |
Volume 12 Issue 3 |
Year of Publication : 2025 |
Authors : Prashant D. Kamble, Shilpa B. Sahare, Heena D. Mehta |
How to Cite?
Prashant D. Kamble, Shilpa B. Sahare, Heena D. Mehta, "Multi-Objective Optimization (MOO) of Machining Process Factors by Hybrid Taguchi Grey Relational Analysis (HGRA)," SSRG International Journal of Mechanical Engineering, vol. 12, no. 3, pp. 109-115, 2025. Crossref, https://doi.org/10.14445/23488360/IJME-V12I3P109
Abstract:
An effort to optimize machining processes, such as turning operations, is made in this work. The following are adjustable process parameters: Cutting Environment (CE), Feed Rate (FR), Depth Of Cut (DOC), Nose Radius (NR), and Type of Tool (TT). The parameters of the response are Surface Roughness (SR), Material Removal Rate (MRR), Cutting Force (CF), Tool tip Temperature (Temp) and Tool Wear (TW). For experiments, the L27 Taguchi (OA) Orthogonal Array is employed. The CNC Spinner lathe was used for the experiment. The most recent coolant system, MQL, is employed in today's manufacturing setting. Taguchi method and Grey Relational Analysis (TGRA) are utilized for data analysis. GRA processes several output parameters and combines them into a single response parameter. Lastly, the ideal process parameters are determined using the traditional Taguchi approach. The contribution and ranking of input parameters are determined using ANOVA and Signal-to-Noise Ratio (SRN) in order to maximize the number of output parameters. The conformance test is used to formulate the results' hypothesis. The conformance test findings showed that both the experimental and projected values are acceptable.
Keywords:
Taguchi method, Turning process, Multi-optimization, Grey Relational Analysis.
References:
[1] Bhanodaya Kiran Babu Nadikudi, “Multi Objective Optimization of Turning Operation Parameters for Aluminium Alloy Using Grey Relational Analysis Method,” Materials Science Forum, vol. 1120, pp. 43-49, 2024.
[CrossRef] [Google Scholar] [Publisher Link]
[2] Fatlume Zhujani et al., “Optimization of Multiple Performance Characteristics for CNC Turning of Inconel 718 Using Taguchi–Grey Relational Approach and Analysis of Variance,” Metals, vol. 14, no. 2, pp. 1-19, 2024.
[CrossRef] [Google Scholar] [Publisher Link]
[3] Subhas Chandra Moi, and Rajeev Ranjan, “Analysis of Chips Geometry and Parametric Optimization using Grey Relational Analysis (GRA) in Hot Turning of AISI 202,” Transdisciplinary Research and Education Center for Green Technologies, Kyushu University, pp. 242-252, 2024.
[CrossRef] [Google Scholar] [Publisher Link]
[4] A. Bahei El-Deen, “Multi Optimization in Slot Milling of CFRP Composites through Grey Relational-Based Taguchi Analysis,” Nigerian Journal of Technological Development, vol. 21, no. 1, pp. 11-18, 2024.
[CrossRef] [Google Scholar] [Publisher Link]
[5] M. Marichamy, and K. Chockalingam, “Process Parameters for Friction Stir Welding on A319 Aluminium Alloy Using Hybrid Technique for Order of Preference by Similarity to an Ideal Solution and Grey Relational Analysis Multi-Criteria Decision-Making Method,” Proceedings of the Institution of Mechanical Engineers, Part E: Journal of Process Mechanical Engineering, vol. 238, no. 2, pp. 945-953, 2023.
[CrossRef] [Google Scholar] [Publisher Link]
[6] R.A. Kazeem et al., “Evaluation of Crude Watermelon Oil as Lubricant in Cylindrical Turning of AISI 1525 Steel Employing Taguchi and Grey Relational Analyses Techniques,” Heliyon, vol. 10, no. 3, pp. 1-29, 2024.
[CrossRef] [Google Scholar] [Publisher Link]
[7] E.S. Alaba et al., “Evaluation of Palm Kernel Oil as Cutting Lubricant in Turning AISI 1039 Steel Using Taguchi-Grey Relational Analysis Optimization Technique,” Advances in Industrial and Manufacturing Engineering, vol. 6, pp. 1-11, 2023.
[CrossRef] [Google Scholar] [Publisher Link]
[8] Sisay Workineh Agebo, Mahaboob Patel, and Mariusz Deja, “Multi-Response Optimization on the Effect of Wet and Eco-Friendly Cryogenic Turning of D2 Steel Using Taguchi-Based Grey Relational Analysis,” The International Journal of Advanced Manufacturing Technology, vol. 128, no. 9-10, pp. 4561-4578, 2023.
[CrossRef] [Google Scholar] [Publisher Link]
[9] Nanang Ali Sutisna, and Sapto Agus Nowoasto, “Optimization of Machining Parameters and Tool Angle on Surface Quality of Turning Operation Using Taguchi Grey Relational Analysis,” ROTASI, vol. 25, no. 2, pp. 1-7, 2023.
[CrossRef] [Google Scholar] [Publisher Link]
[10] Hongyan Wang et al., “A Systematic Review of Precision Mechanical Machining Processes for Monocrystalline Silicon Based on Modeling And Simulation,” The International Journal of Advanced Manufacturing Technology, vol. 136, pp. 4715-4749, 2025.
[CrossRef] [Google Scholar] [Publisher Link]
[11] Soumikh Roy et al., “Exploring the Viability of Alternative Cooling-Lubrication Strategies in Machining Processes: A Comprehensive Review on the Performance and Sustainability Assessment,” Proceedings of the Institution of Mechanical Engineers, Part B: Journal of Engineering Manufacture, vol. 239, no. 3, pp. 307-349, 2025.
[CrossRef] [Google Scholar] [Publisher Link]
[12] Syed Altaf Hussain et al., “Turning GFRP Composites with Multi-Response Optimisation Using TOPSIS Method,” International Journal on Interactive Design and Manufacturing, vol. 19, no. 2, pp. 1327-1339, 2025.
[CrossRef] [Google Scholar] [Publisher Link]