A novel SVM neural network based clinical diagnosis of cardiac rhythm
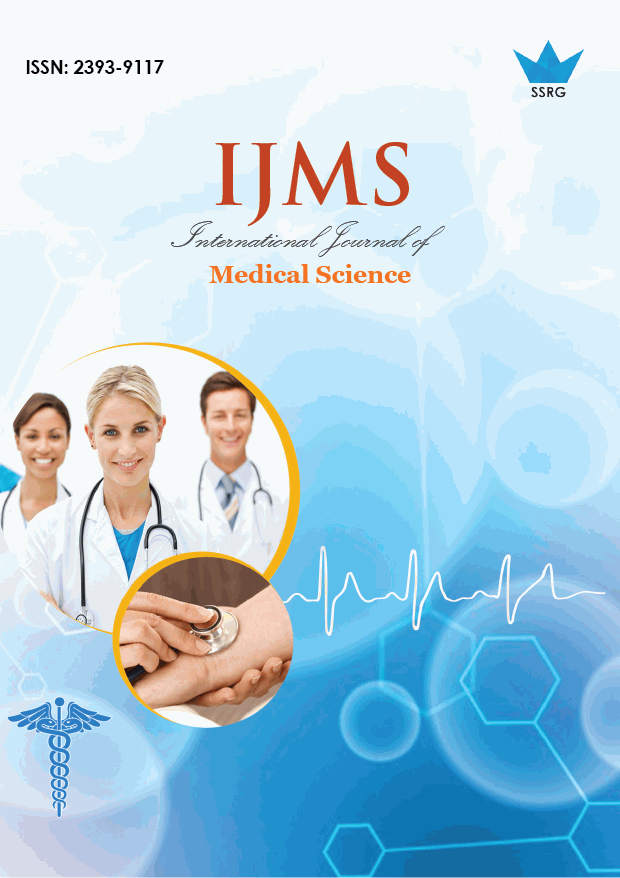
International Journal of Medical Science |
© 2017 by SSRG - IJMS Journal |
Volume 4 Issue 1 |
Year of Publication : 2017 |
Authors : S.Arivoli |
How to Cite?
S.Arivoli, "A novel SVM neural network based clinical diagnosis of cardiac rhythm," SSRG International Journal of Medical Science, vol. 4, no. 1, pp. 8-14, 2017. Crossref, https://doi.org/10.14445/23939117/IJMS-V4I1P103
Abstract:
The cardiovascular disease is one of main disease that threatens the human life. There are many ways to find cardiac arrhythmia. Electrocardiogram (ECG) is a key diagnostic tool to monitor and visualize the electrical activity of heart and is used to study its normal or abnormal functioning. Physicians perform routine diagnosis the functioning of heart by visually examining the shapes of ECG waveform. However, automatic processing and classification of ECG data would be extremely useful in patient monitoring and telemedicine systems. Such real time applications require techniques that are highly accurate and very efficient. A new method for arrhythmia classification for clinical diagnosis is proposed in this project.ECG signal being one of the most analyzed for well being humans. The analysis of electrical activity of heart is done on ambulatory for ICU.For diagnosis, efficient methods are required to classify and exact arrhythmia. In this paper we are proposing a new method and approach by which the ECG arrhythmia is classified based on support vector machine (SVM).
Keywords:
Electrocardiogram, support vector machine, arrhythmia.
References:
[1] Chinmay Chandrakar, M.K.Kowar, “Denoising ECG Signals using Adaptive Filter Algorithm” , International Journal of Soft Computing and Engineering (IJSCE), March 2012.
[2] Chao Lin, “P and T wave Detection of ECG signals”, International Journal of Modern Engineering Research (IJMER), June 2011.
[3] Soumya Ranjan Mishra & K.Goutham, “Real time classification of ECG waveforms for diagnosis of diseases”, IEEE Transaction on Biomedical Engineering, 2009-10.
[4] S.S.Mehta, N.S.Lingayat, “Identification of QRS complex in 12-lead ECG”, Science Direct, Expert Systems with Applications, May 2007.
[5] C.Li, C.Zheng et.al, “Detection of ECG characteristics points using wavelet transform”, IEEE Transaction on Biomedical Engineering, 2001.
[6] Osowki. S., & Linh, T.H., “ECG beat recognition using fuzzy hybrid neural network”, IEEE Transaction on Biomedical Engineering, 2001.
[7] Jiapu Pan and Willis J.Tompkins, “A Real Time QRS Detection Algorithm”, IEEE Transaction on Biomedical Engineering, 1985.