Predictive Model for Corrosion Rate of Mild Steel using Artificial Neural Network
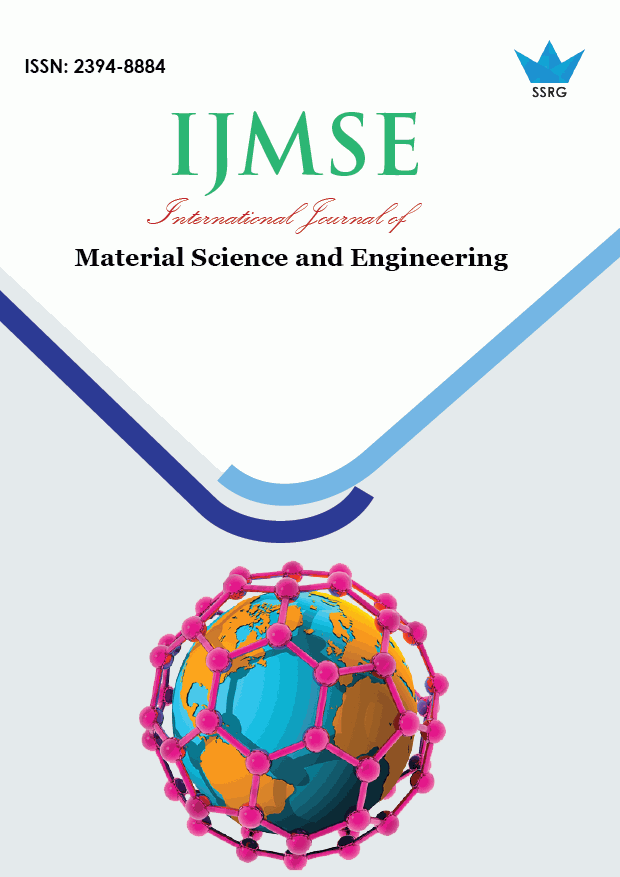
International Journal of Material Science and Engineering |
© 2023 by SSRG - IJMSE Journal |
Volume 9 Issue 3 |
Year of Publication : 2023 |
Authors : Abdussalam Mamoon, Abdul Audu, Nazir Nasir Yunusa |
How to Cite?
Abdussalam Mamoon, Abdul Audu, Nazir Nasir Yunusa, "Predictive Model for Corrosion Rate of Mild Steel using Artificial Neural Network," SSRG International Journal of Material Science and Engineering, vol. 9, no. 3, pp. 1-6, 2023. Crossref, https://doi.org/10.14445/23948884/IJMSE-V9I3P101
Abstract:
The potential of using an Artificial Neural Network (ANN) in predicting the corrosion rate of mild steel exposed to five corrosive environments has been studied in the present work. The corrosion rate of mild steel exposed to five different environments, namely, hydrochloric acid, alkaline solution, natural seawater, freshwater, and pasty soil, using the laboratory immersion test method, has been investigated. Three sets of samples were prepared and exposed, each for corrosion rate tests across the five (5) prepared polyethylene-sealed environments. At an interval of four weeks, samples were collected, observed, cleaned, and subjected to corrosion rate tests for twenty weeks. The experimental results of the corrosion rate data obtained by the weight loss method were used to create a database for training and testing the feedforward backpropagation NN model. The result shows the training R-value of 0.99983, validation R-value of 0.99956, test R-value of 0.99562, and the overall Rvalue of 0.99981. This indicates that the experimental data agrees with the simulated data with minimum difference. Moreover, the result proves that the developed model and the network training, testing, and validation procedure are significantly acceptable. Hence, the validation of the proposed ANN agrees with the actual experimental results. This shows that the ANN model could be attractive as a corrosion rate estimator.
Keywords:
ANN, Corrosion, Mild steel, Model, Predictive model.
References:
[1] Harish Kumar, and Vikas Yadav, “Corrosion Characteristics of Mild Steel under Different Atmospheric Conditions by Vapour Phase Corrosion Inhibitors,” American Journal of Materials Science and Engineering, vol. 1, no. 3, pp. 34-39, 2018.
[Google Scholar] [Publisher Link]
[2] Kashmitha Muthamma et al., “Corrosion Inhibition of Mild Steel in Acidic Media by N-[(3,4-Dimethoxyphenyl) Methyleneamino]-4- Hydroxy-Benzamide,” Journal of Bio-and Tribo-Corrosion, vol. 7, 2021.
[CrossRef] [Google Scholar] [Publisher Link]
[3] B. Anand, and V. Balasubramanian, “Corrosion Behaviour of Mild Steel in Acidic Medium in Presence of Aqueous Extract of Allamanda Blanchetii,” Journal of Chemistry, vol. 8, no. 1, pp. 226–230, 2010.
[CrossRef] [Google Scholar] [Publisher Link]
[4] Ahmed A. Al-Amiery et al., “Experimental and Theoretical Study on the Corrosion Inhibition of Mild Steel by Nonanedioic Acid Derivative in Hydrochloric Acid Solution,” Scientific Reports, vol. 12, no. 1, 2022.
[CrossRef] [Google Scholar] [Publisher Link]
[5] A.M. Fekry, and M.A. Ameer, “Corrosion Inhibition of Mild Steel in Acidic Media using Newly Synthesized Heterocyclic Organic Molecules,” International Journal of Hydrogen Energy, vol. 35, no. 14, pp. 7641–7651, 2010.
[CrossRef] [Google Scholar] [Publisher Link]
[6] Amir Akhtari-Goshayeshi et al., “Prediction of Corrosion Rate for Carbon Steel in Soil Environment by Artificial Neural Network and Genetic Algorithm,” Journal of Advanced Materials and Processing, vol. 7, no. 4, pp. 30-43 2019.
[Google Scholar] [Publisher Link]
[7] Mst. Kamrunnahar, and Mirna Urquidi-Macdonald, “Prediction of Corrosion Behavior of Alloy 22 Using a Neural Network as a Data Mining Tool,” Corrosion Science, vol. 53, no. 3, pp. 961–967, 2011.
[CrossRef] [Google Scholar] [Publisher Link]
[8] E. Osarolube, I. Owate, and N.C. Oforka, “Corrosion Behavior of Mild and High Carbon Steels in Various Acidic Media,” Scientific Research and Essays, vol. 3, pp. 224–228, 2008.
[Google Scholar] [Publisher Link]
[9] B.O. Odio et al., “Investigation of the Effect of Corrosion on Mild Steel in Five Different Environments,” International Journal of Scientific & Technology Research, vol. 3, no. 7, pp. 306-310, 2014.
[Google Scholar] [Publisher Link]
[10] A. Mamoon, N. Y. Nasir, and A. G. Musa, “Investigation of the Effect of Corrosion on the Mechanical Properties of Mild Steel Exposed to Five Different Environments,” Journal of the Nigerian Institution of Mechanical Engineers, vol. 8, no. 2, pp. 88–95, Sep. 2018.
[11] C.I. Rocabruno-Valdés et al., “Corrosion Rate Prediction for Metals in Biodiesel Using Artificial Neural Networks,” Renewable Energy, vol. 140, pp. 592–601, 2019.
[CrossRef] [Google Scholar] [Publisher Link]
[12] Deden Supriyatman et al., “Artificial Neural Networks for Corrosion Rate Prediction in Gas Pipelines,” SPE Annual Technical Conference and Exhibition, vol. 2, 2012.
[CrossRef] [Google Scholar] [Publisher Link]
[13] Hsiang-Teng Lin, Chien-Ming Lo, and Min-Der Lin, “Application of Artificial Neural Networks on Predicting Corrosion Rates of Carbon Steel in Taiwan Industrial Zones,” Advances in Intelligent Systems Research, 2017.
[CrossRef] [Google Scholar] [Publisher Link]
[14] Mohammed Hayajneh et al., “Prediction of tribological Behavior of Aluminum–Copper-Based Composite sing Artificial Neural Network,” Journal of Alloys and Compounds, vol. 470, no. 1-2, pp. 584–588, 2009.
[CrossRef] [Google Scholar] [Publisher Link]
[15] Mst. Kamrunnahar, and Mirna Urquidi-Macdonald, “Prediction of Corrosion Behavior Using a Neural Network as a Data Mining Tool,” Corrosion Science, vol. 52, no. 3, pp. 669–677, 2010.
[CrossRef] [Google Scholar] [Publisher Link]
[16] P. Prudhvi Sai et al., “Artificial Neural Network Models for Predicting the Corrosion Behavior of Friction Stir Processed AA5083,” Materials Today: Proceedings, vol. 46, pp. 7215–7219, 2021.
[CrossRef] [Google Scholar] [Publisher Link]
[17] X. Xia et al., “An Artificial Neural Network for Predicting Corrosion Rate and Hardness of Magnesium Alloys,” Materials & Design, vol. 90, pp. 1034–1043, 2016.
[CrossRef] [Google Scholar] [Publisher Link]
[18] Elaine D. Kenny et al., “Artificial Neural Network Corrosion Modeling for Metals in an Equatorial Climate,” Corrosion Science, vol. 51, no. 10, pp. 2266–2278, 2009.
[CrossRef] [Google Scholar] [Publisher Link]
[19] Haodong Ji, and Hailong Ye, “Machine Learning Prediction of Corrosion Rate of Steel in Carbonated Cementitious Mortars,” Cement and Concrete Composites, vol. 143, 2023.
[CrossRef] [Google Scholar] [Publisher Link]
[20] Qiang Li et al., “Modeling the Corrosion Rate of Carbon Steel in Carbonated Mixtures of MDEA-Based Solutions Using Artificial Neural Network,” Process Safety and Environmental Protection, vol. 147, pp. 300–310, 2021.
[CrossRef] [Google Scholar] [Publisher Link]